Ensuring clean, consistent, and accurate data is fundamental to the success of any clinical trial, and lab data reconciliation sits at the heart of this effort. As clinical trials become more complex and data sources more diverse, the need for a streamlined and precise process has never been greater. Without effective oversight, inconsistencies can delay trial timelines, raise compliance concerns, and compromise patient safety.
aRead on as this article dives into how to improve lab data reconciliation in clinical data management and reduce the risk of costly discrepancies.
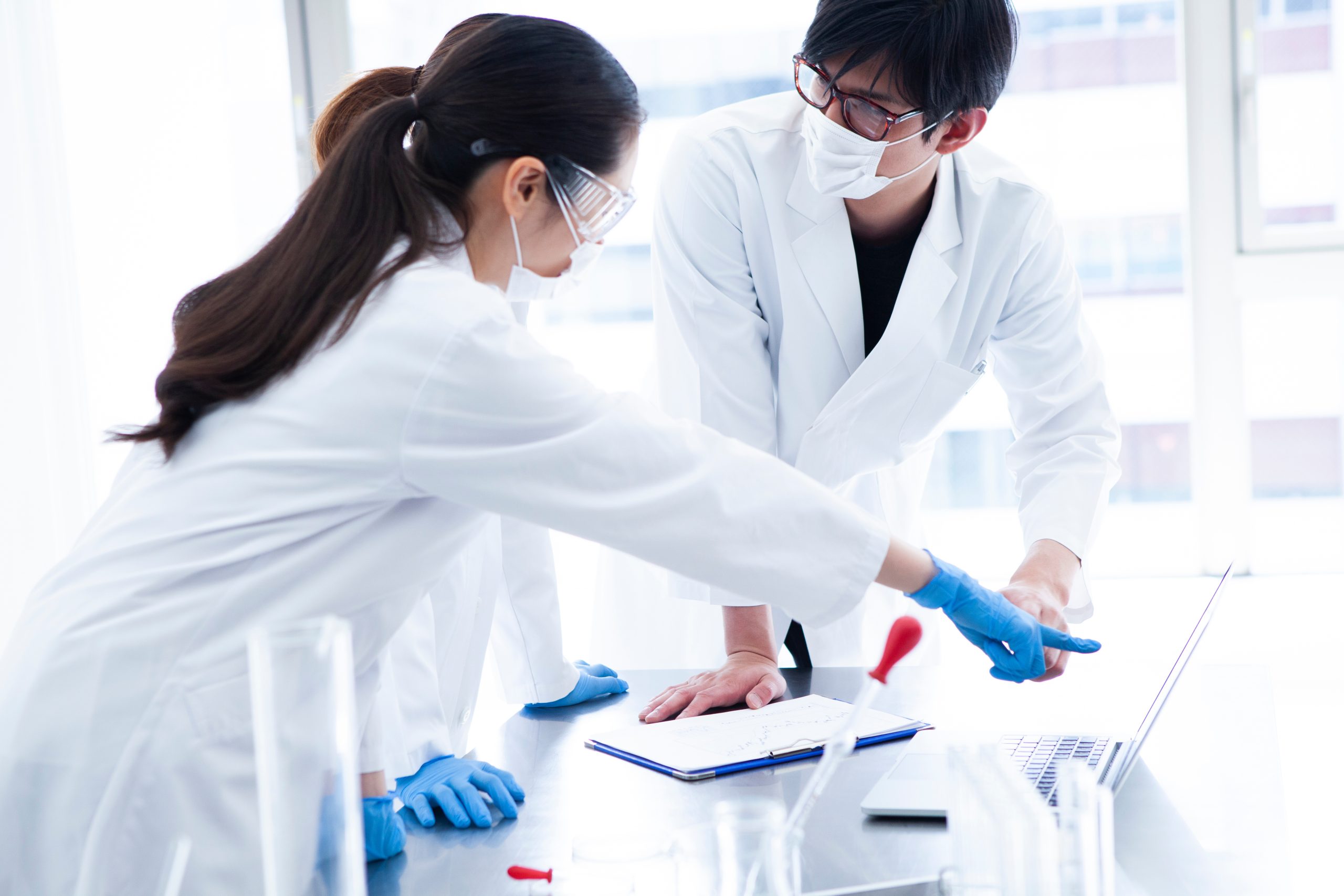
Work with Experienced Clinical Data Partners and Leverage Specialized Tools
Partnering with experienced professionals can bring clarity and control to a complex clinical data reconciliation process. When internal resources are stretched or lack deep familiarity with reconciliation challenges, partners provide valuable operational support. Their exposure to diverse clinical studies can also enable them to apply proven strategies that improve consistency and reduce reconciliation delays.
In addition to technical skill, they often bring process maturity. They understand how to align timelines, manage site variability, and work with multiple data formats. They also use specialized data reconciliation tools, which can automate comparison processes, flag discrepancies through algorithms, and maintain comprehensive audit trails—capabilities that generic spreadsheet solutions cannot match.
All these become particularly useful when managing studies involving several central or specialty labs. Rather than spending time correcting formatting or reconciling structural mismatches, your internal team can focus on high-value clinical oversight.
Moreover, seasoned partners typically stay aligned with current industry trends and regulatory expectations. They’re familiar with global standards and common audit findings, which helps reduce compliance risks. This knowledge allows them to anticipate reconciliation issues before they surface and ensure that your data is well-positioned for submission.
Establish Clear Data Standards
Before any meaningful reconciliation can begin, establishing clear and consistent data standards is essential. Inconsistent formats across clinical and lab data can slow down efforts and introduce discrepancies that are difficult to trace. But setting expectations for uniformity early on creates a foundation that supports cleaner data and more efficient processes throughout the study.
To start, using industry-recognized coding systems is critical. Implementing standards like MedDRA for adverse event terms and lab-related parameters allows teams to speak the same language across datasets. This reduces the risk of interpretation errors and simplifies the comparison of data from multiple sources.
Beyond coding systems, it’s important to align how basic data elements are recorded. Units of measure, for example, should follow a consistent structure across all systems to avoid confusion during reconciliation. Similarly, standardizing reference ranges helps eliminate mismatches when reviewing lab results against clinical parameters.
These small details can have a large impact on how smoothly datasets align later in the process.
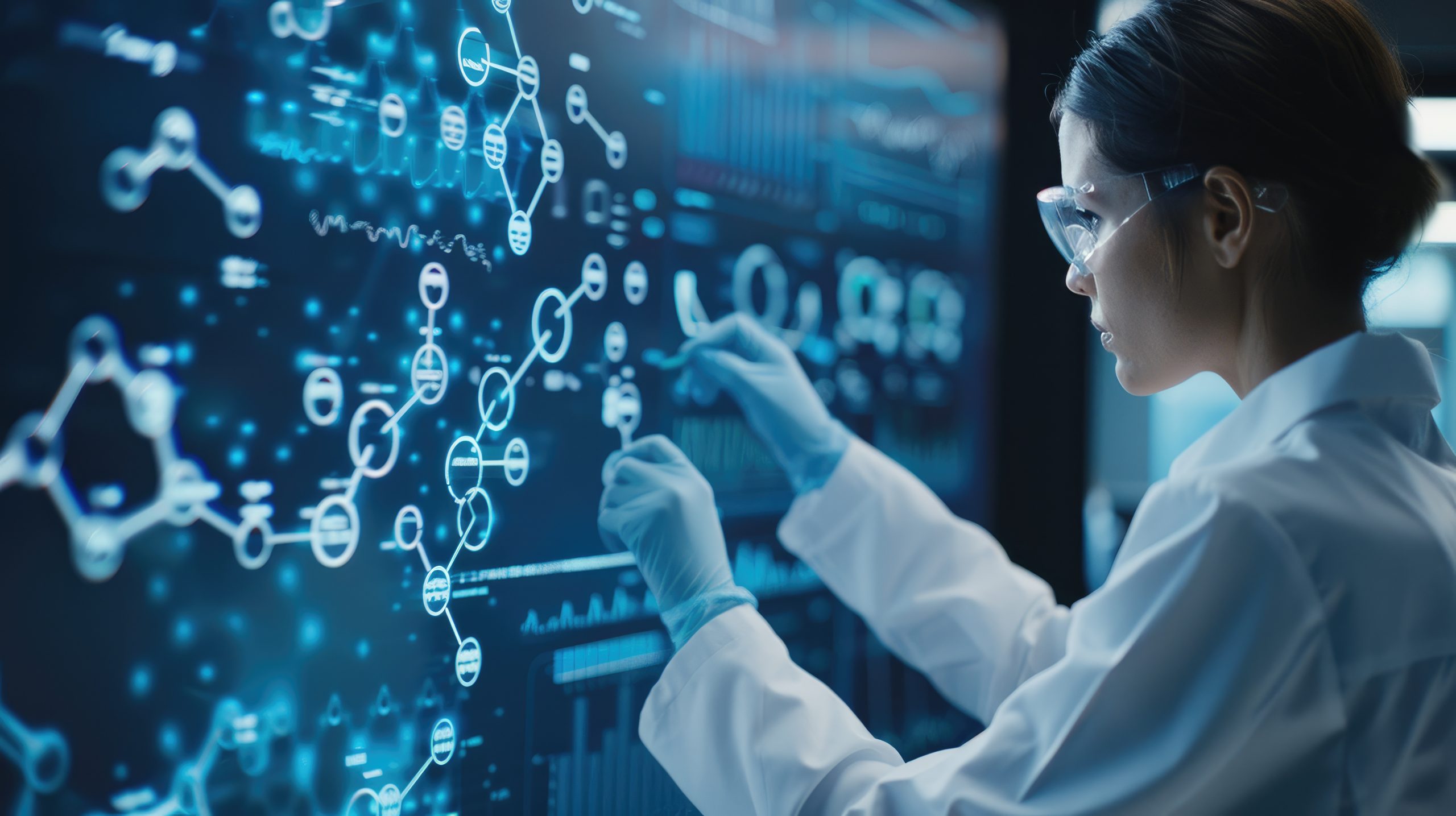
Strengthen Coordination with External Vendors
External labs and third-party providers often follow their own data collection processes, which can cause reconciliation delays and data discrepancies. That said, establishing clear communication channels from the outset helps reduce misinterpretation and ensures everyone is working from the same expectations.
Also, in today’s healthcare system, where data comes from a variety of sources, creating alignment through well-defined documentation is even more crucial. Standard operating procedures should clearly outline each party’s responsibilities, especially during data handoffs and reconciliation milestones. These procedures serve as a shared reference, reducing confusion when issues arise. A documented framework also promotes accountability and makes it easier to train new team members or onboard new vendors mid-study.
As the trial progresses, it becomes increasingly important to keep vendors engaged with your data requirements. Requesting structured data in predefined formats can help prevent formatting issues during ingestion into your clinical data management system. And when vendors understand exactly how their data needs to be delivered, the risk of rework or compatibility challenges decreases.
Integrate Automated Validation Checks
Automating validation checks is one of the most effective ways to reduce the manual workload in lab data reconciliation. While manual reviews are still important for resolving complex discrepancies, relying solely on human effort can slow down the process and increase the risk of oversight. Automated tools help flag issues early and keep the data review cycle on track.
Set up targeted checks that evaluate specific parameters to catch errors that would otherwise slip through. These can include logic-based validations, such as confirming that lab values align with the protocol’s expected ranges, or consistency checks that compare dates, test names, and result patterns for anomalies. When configured correctly, these rules can run across large data sets quickly, alerting data managers to areas that require closer review.
In addition to flagging discrepancies, automated validation can highlight data gaps. For example, missing lab results or test codes that do not match expected inputs can be identified in near real-time. This makes it easier to follow up with vendors or site teams before data locks or submission deadlines approach. Automating this layer of quality control can lead to fewer delays caused by incomplete datasets.
Validate Before Final Lock
Once the majority of reconciliation activities are completed, the focus must shift to a thorough verification of all data elements that impact reporting and submission. This includes ensuring that all non-CRF data, such as laboratory results, are fully aligned with entries in the electronic data capture system and cross-checked against the safety database.
To support this, a structured and traceable review process must be in place. Start with a final assessment of data across all systems to detect mismatches in collection dates, missing subject identifiers, or unresolved mismatches in adverse events. Each issue should have documented resolution steps logged in the comprehensive audit trail.
Next, confirm that all protocol-specified parameters are accounted for, especially those that directly influence clinical trial outcomes or are tied to secondary endpoints. This step often requires a coordinated review between the CDM team, clinical leads, and statistical reviewers to ensure nothing has been misclassified or omitted.
Final Thoughts
A strong lab data reconciliation process doesn’t just support compliance—it reflects a deeper commitment to data integrity and scientific responsibility. In an environment where every data point can influence regulatory decisions and patient outcomes, how you manage discrepancies speaks volumes about the quality of your trial oversight.
Prioritize clarity, consistency, and proactive problem-solving, and you can strengthen the foundation of your entire clinical data management strategy.
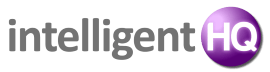
Founder Dinis Guarda
IntelligentHQ Your New Business Network.
IntelligentHQ is a Business network and an expert source for finance, capital markets and intelligence for thousands of global business professionals, startups, and companies.
We exist at the point of intersection between technology, social media, finance and innovation.
IntelligentHQ leverages innovation and scale of social digital technology, analytics, news, and distribution to create an unparalleled, full digital medium and social business networks spectrum.
IntelligentHQ is working hard, to become a trusted, and indispensable source of business news and analytics, within financial services and its associated supply chains and ecosystems