Artificial intelligence is changing the game in many fields, especially with the rise of agenting AI. These smart systems are designed to perform tasks and make decisions on their own, which can lead to huge improvements in how we work and interact with technology. In this article, we’ll break down what agenting AI is, how it functions, and its impact across various industries.
Key Takeaways
- Agenting AI refers to autonomous programs that can perform tasks without constant human input.
- These systems use machine learning to analyze data and make decisions in real time.
- Industries like customer service, healthcare, and finance are seeing major benefits from agenting AI.
- While there are many advantages, challenges like data privacy and algorithmic bias must be addressed.
- The future of agenting AI looks promising, with trends pointing towards smarter, more autonomous systems.
Understanding Agenting AI
Agenting AI is really changing how we think about automation. It’s not just about following rules anymore; it’s about creating systems that can actually think and act on their own. Let’s break down what this means.
Defining Agenting AI
So, what exactly is Agenting AI? Well, at its core, it’s about building AI systems that can perceive their environment, make decisions, and take actions to achieve specific goals. The key here is autonomy. Unlike traditional AI, which often requires constant human oversight, Agenting AI is designed to operate independently. Think of it like this: a regular program follows a script, but an AI agent writes its own script as it goes along. For example, NVIDIA Llama Nemotron is a platform designed to enhance reasoning capabilities in agentic AI systems.
How Agenting AI Works
Agenting AI typically works through a cycle of perception, reasoning, and action. Here’s a simplified view:
- Perception: The agent gathers information from its environment using sensors or data inputs.
- Reasoning: It processes this information, using machine learning models to understand patterns and predict outcomes.
- Decision-Making: Based on its analysis, the agent chooses the best course of action.
- Action: The agent executes the chosen action, which then affects the environment, starting the cycle anew.
Agenting AI mimics human intelligence but operates at much higher speeds, analyzing vast amounts of information in real time. The ability to act decisively on its decisions is what truly distinguishes agentic AI from traditional AI systems.
The Role of Machine Learning
Machine learning is the engine that drives Agenting AI. It allows agents to learn from data, adapt to changing conditions, and improve their performance over time. Without machine learning, AI agents would be stuck with pre-defined rules and unable to handle the complexities of the real world. Machine learning models help agents understand patterns, make predictions, and optimize their actions to achieve their goals. For example, machine learning models help agents understand patterns, make predictions, and optimize their actions to achieve their goals. This is how AI agents are transforming industries.
Applications of Agenting AI
Transforming Customer Service
Agenting AI is changing customer service in a big way. Think about those times you’re stuck waiting forever to talk to someone – AI can help. AI-powered chatbots customer service chatbots can handle many common questions instantly, freeing up human agents for more complex issues. It’s like having a super-efficient first line of support that’s available 24/7. This means faster response times and happier customers. Plus, AI can analyze customer interactions to find areas where service can be improved. It’s not just about answering questions; it’s about making the whole experience better.
- Instant responses to common inquiries
- 24/7 availability
- Personalized support based on customer history
Agenting AI in customer service isn’t just about replacing humans; it’s about helping them. By taking care of routine tasks, AI allows human agents to focus on the more complicated and sensitive issues that require a personal touch. This leads to a more efficient and effective customer service operation overall.
Enhancing Healthcare Solutions
Healthcare is another area where Agenting AI is making a real difference. From diagnosing diseases to managing patient data, AI can help doctors and nurses provide better care. For example, AI can analyze medical images to detect early signs of cancer, or it can monitor patients’ vital signs to predict potential health problems. It’s like having an extra set of eyes and ears that never get tired. And because AI can process huge amounts of data, it can help researchers find new treatments and cures for diseases. It’s not a replacement for human doctors, but it’s a powerful tool that can help them save lives.
- Improved accuracy in diagnoses
- Personalized treatment plans
- Faster drug discovery
Revolutionizing Financial Services
In the world of finance, Agenting AI is being used to automate tasks, detect fraud, and provide personalized financial advice. Imagine an AI that can analyze your spending habits and suggest ways to save money, or one that can detect suspicious transactions and prevent fraud. That’s the power of Agenting AI in financial services. It can also be used to automate trading, manage risk, and provide customer support. It’s like having a personal financial advisor that’s available 24/7. And because AI can process huge amounts of data, it can help financial institutions make better decisions and stay ahead of the curve. AI agents AI agents are reshaping industries by enhancing efficiency, decision-making, and scalability.
- Automated fraud detection
- Personalized financial advice
- Improved risk management
Feature | Traditional Method | Agenting AI Method | Benefit |
---|---|---|---|
Fraud Detection | Manual review | AI-powered analysis | Faster detection, reduced false positives |
Risk Assessment | Limited data | Comprehensive data | More accurate risk assessment |
Customer Service | Human agents | AI chatbots | 24/7 availability, instant responses |
Benefits of Implementing Agenting AI
Agenting AI is making waves, and for good reason. It’s not just about the hype; there are real, tangible benefits to bringing these systems into your operations. Let’s break down some of the key advantages.
Increased Efficiency
Agenting AI really shines when it comes to boosting efficiency. These systems can automate tasks at speeds humans simply can’t match. Think about it: repetitive data entry, sifting through customer inquiries, or monitoring system performance. Agenting AI can handle all of that, freeing up your human employees to focus on more strategic, creative, and complex work. This not only speeds up processes but also reduces the chance of errors that can creep in with manual work. It’s like having a tireless, super-efficient assistant who never needs a coffee break. For example, AI-driven automation can significantly reduce the time it takes to complete tasks.
Improved Accuracy
Speaking of errors, accuracy is another major win with agenting AI. These systems are designed to process information and execute tasks with a high degree of precision. They don’t get tired, distracted, or make mistakes based on gut feelings. Instead, they rely on data and algorithms to make decisions, leading to more consistent and reliable outcomes. This is especially important in fields like finance and healthcare, where even small errors can have big consequences. Think about fraud detection, medical diagnoses, or financial forecasting – agenting AI can bring a new level of accuracy to these critical areas. The use of AI agents improves real-time assistance and personalized service.
Cost Reduction Strategies
Ultimately, all these benefits add up to significant cost savings. By automating tasks, reducing errors, and improving efficiency, agenting AI can help organizations streamline their operations and lower their expenses. This can involve reducing the need for manual labor, minimizing waste, and optimizing resource allocation. For example, agenting AI can analyze energy consumption patterns and adjust settings to reduce utility bills, or it can optimize supply chains to minimize inventory costs. It’s about finding those areas where automation can make the biggest impact and then implementing agenting AI to drive down costs.
Agenting AI isn’t just about cutting costs; it’s about making smarter investments. By freeing up resources and improving efficiency, organizations can reinvest in innovation, growth, and other strategic initiatives. It’s a shift from simply doing things cheaper to doing things better.
Challenges in Agenting AI Deployment
Agenting AI sounds amazing, right? But getting it up and running isn’t always a walk in the park. There are definitely some hurdles to consider before you jump in. Let’s talk about some of the main challenges you might face.
Data Privacy Concerns
Okay, so agenting AI thrives on data. The more data it has, the better it performs. But here’s the thing: all that data comes with a huge responsibility. You’ve got to make sure you’re protecting people’s information like it’s Fort Knox. Think about it – if sensitive data gets into the wrong hands, it could be a disaster. We’re talking about serious breaches of trust and potential legal nightmares. So, what can you do?
- Implement strong encryption methods to protect data both when it’s being stored and when it’s being transmitted.
- Make sure you’re following all the data privacy regulations, like GDPR or CCPA. It’s not just a good idea; it’s the law.
- Regularly audit your systems to find and fix any vulnerabilities. Think of it as giving your digital defenses a check-up.
It’s important to remember that data privacy isn’t just a technical issue; it’s an ethical one. People need to trust that their data is safe, or they won’t use your services. Building that trust is key to the long-term success of any agenting AI project.
Algorithmic Bias Issues
AI is only as good as the data it learns from. If that data reflects existing biases, the AI will, too. This can lead to some seriously unfair or discriminatory outcomes. Imagine an AI used for hiring that favors one group of people over another, or a financial advisor robot that gives different advice based on someone’s race. Not cool, right?
So, how do you tackle this? Here’s a start:
- Carefully examine the data you’re using to train your AI. Look for any signs of bias and correct them.
- Test your AI systems rigorously to see if they’re producing biased results. Get a diverse group of people to help with this.
- Be transparent about how your AI systems work. Explain how decisions are made and what data is used.
Integration with Existing Systems
Trying to fit agenting AI into your current setup can be like trying to shove a square peg into a round hole. A lot of companies have older systems that just weren’t designed to work with AI. This can lead to all sorts of headaches, like compatibility issues, data silos, and just plain old frustration. Here’s a few things to keep in mind:
- Assess your current systems to see how well they’ll work with AI. You might need to upgrade or replace some of them.
- Plan for a phased rollout of AI. Don’t try to do everything at once. Start with a small project and gradually expand from there.
- Make sure your IT team has the skills and knowledge they need to support AI. If not, invest in training or hire some experts.
| Challenge | Description the robot financial advisors.
Future Trends in Agenting AI
Agenting AI is moving fast! It’s not just about automating simple tasks anymore. We’re talking about AI that can really think and act on its own. Let’s look at some of the big things coming up.
Advancements in Natural Language Processing
NLP is getting way better, and that’s a game-changer for agenting AI. AI agents will understand us better than ever. They’ll be able to handle complex requests, understand context, and even pick up on emotions. Think about how much easier it will be to interact with AI when it feels like talking to another person. This will improve diagnostics and make everything smoother.
The Rise of Autonomous Agents
We’re going to see more and more AI agents that can operate completely on their own. These agents won’t just follow instructions; they’ll be able to set their own goals, plan their own actions, and learn from their experiences. Imagine AI agents managing entire supply chains, conducting scientific research, or even exploring new planets – all without needing constant human supervision. It sounds like science fiction, but it’s closer than you think.
Impact on Workforce Dynamics
Agenting AI will change how we work, no doubt. Some jobs will be automated, but new jobs will also be created. It’s all about adapting and learning new skills. We’ll need people who can train AI agents, manage AI systems, and work alongside AI to solve complex problems. The key is to see AI as a tool that can help us be more productive and creative, not as a replacement for human workers.
The shift towards agenting AI requires a proactive approach to workforce development. Investing in education and training programs will be essential to prepare workers for the jobs of the future. This includes teaching skills in AI development, data analysis, and human-machine collaboration.
Here’s a quick look at how different job sectors might change:
Sector | Impact |
---|---|
Customer Service | More AI-powered chatbots and virtual assistants |
Healthcare | AI-assisted diagnostics and patient care |
Finance | Automated trading and fraud detection |
Ethical Considerations in Agenting AI
Agenting AI is cool, but it’s not all sunshine and rainbows. We need to think about the ethical stuff too. It’s easy to get caught up in the tech and forget that these systems can have a real impact on people’s lives. So, let’s talk about some of the big ethical questions that come up with agenting AI.
Ensuring Transparency
One of the biggest problems is that agenting AI can be a black box. You put data in, and it spits out a decision, but it’s not always clear why it made that decision. This lack of transparency can be a real issue, especially when the AI is making important calls. We need to find ways to make these systems more transparent so we can understand how they work and why they make the choices they do.
Think about it: if an AI denies someone a loan, that person deserves to know why. If we don’t have transparency, it’s hard to trust the AI, and it’s hard to fix problems when they come up. One way to improve transparency is to use explainable AI (XAI) techniques. XAI aims to make AI decisions more understandable to humans. It’s not a perfect solution, but it’s a step in the right direction. Another thing is to make sure the data used to train the AI is well-documented and that the AI’s decision-making process is auditable. This way, if something goes wrong, we can trace it back to the source.
Addressing Accountability
So, who’s to blame when an agenting AI messes up? Is it the programmer? The company that deployed the AI? The AI itself? This is a tough question, and there’s no easy answer. But we need to figure out who’s accountable because, without accountability, there’s no incentive to make sure these systems are used responsibly.
One approach is to establish clear lines of responsibility. For example, the company that deploys the AI could be held responsible for its actions, even if they didn’t directly program it. This would incentivize companies to carefully test and monitor their AI systems. Another approach is to create an independent oversight body that can investigate AI-related incidents and recommend corrective actions. This body could also help develop ethical guidelines for agenting AI.
Promoting Fairness in AI Systems
AI systems are only as good as the data they’re trained on. If the data is biased, the AI will be biased too. This can lead to unfair or discriminatory outcomes. For example, an AI used for hiring might discriminate against women or minorities if it’s trained on data that reflects past biases. We need to make sure that AI systems are fair and don’t perpetuate existing inequalities. One way to do this is to carefully curate the data used to train the AI. This means making sure the data is representative of the population the AI will be used on and that it doesn’t contain any biases. Another thing is to use techniques to detect and mitigate bias in AI systems. There are a number of these techniques, and they’re constantly evolving. For example, you can use AI developers to help you with this.
Here’s a quick look at some common biases and how to address them:
| Bias Type | Description | Mitigation Strategy </div>
Comparing Agenting AI with Traditional Automation
Key Differences
Traditional automation, like your old-school assembly line robots, follows a script. It’s great for doing the same thing over and over, but it’s not so hot when things change. Agenting AI, on the other hand, is like having a smart assistant that can think on its feet. The biggest difference is that Agenting AI can adapt and learn, while traditional automation just does what it’s told.
Think of it this way:
- Traditional automation: A pre-programmed coffee machine. It makes the same coffee every time, no matter what.
- Agenting AI: A barista that learns your preferences and suggests new drinks based on your mood and the weather. It can even order more beans when they’re running low.
- Agenting AI can automate complex, multi-step processes that previously required extensive human intervention.
Advantages of Agenting AI
Agenting AI brings a lot to the table that traditional automation just can’t match. For starters, it’s way more flexible. It can handle unexpected situations and make decisions on its own. Plus, it’s always learning and improving, so it gets better over time. This means you can use it for things that are too complicated or unpredictable for traditional automation. Businesses can stay ahead by using AI-driven automation.
Here’s a quick rundown:
- Adaptability: Agenting AI can adjust to changing conditions, while traditional automation is rigid.
- Learning: Agenting AI learns from data and experience, improving its performance over time.
- Decision-making: Agenting AI can make decisions autonomously, while traditional automation requires human input for anything outside its pre-programmed instructions.
Limitations of Traditional Automation
While traditional automation is still useful for some things, it has some pretty big limitations. It’s not very flexible, it can’t learn, and it can only do what it’s been programmed to do. This means it’s not a good fit for tasks that require creativity, problem-solving, or adaptability. Plus, it can be expensive and time-consuming to reprogram traditional automation systems when things change. Traditional automation is like a one-trick pony – good at that one trick, but not much else. Consider the benefits of AI agents for more complex tasks.
Traditional automation is great for simple, repetitive tasks, but it falls short when it comes to complex, dynamic environments. Agenting AI offers a more intelligent and adaptable solution for businesses looking to automate a wider range of processes.
Wrapping Up: The Impact of AI Agents
In summary, AI agents are changing the game for how we think about automation and technology. They help businesses streamline tasks, reduce errors, and make smarter decisions. While there are challenges to consider, like privacy and fairness, the potential benefits are huge. As we move forward, it’s clear that AI agents will play a big role in shaping the future of work and everyday life. Embracing this technology can lead to a more efficient and innovative world, making it an exciting time to be involved in the digital landscape.
Frequently Asked Questions
What is Agenting AI?
Agenting AI refers to smart computer programs that can perform tasks on their own, learn from experience, and make decisions without needing constant help from people.
How does Agenting AI work?
Agenting AI works by using data and algorithms to understand information, learn from it, and then use that knowledge to complete tasks or make suggestions.
What are some uses of Agenting AI?
Agenting AI can be used in many areas like customer service, healthcare, and finance to help automate tasks, improve services, and analyze data.
What are the benefits of using Agenting AI?
Benefits include saving time, reducing mistakes, cutting costs, and making processes more efficient.
What challenges does Agenting AI face?
Challenges include keeping data safe, avoiding bias in decision-making, and making sure it works well with existing systems.
What is the future of Agenting AI?
The future of Agenting AI looks bright with advancements in understanding language, creating smarter agents, and changing how people work with technology.
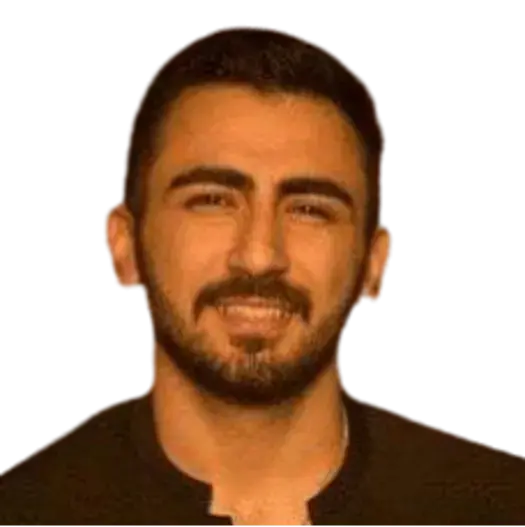
Peyman Khosravani is a seasoned expert in blockchain, digital transformation, and emerging technologies, with a strong focus on innovation in finance, business, and marketing. With a robust background in blockchain and decentralized finance (DeFi), Peyman has successfully guided global organizations in refining digital strategies and optimizing data-driven decision-making. His work emphasizes leveraging technology for societal impact, focusing on fairness, justice, and transparency. A passionate advocate for the transformative power of digital tools, Peyman’s expertise spans across helping startups and established businesses navigate digital landscapes, drive growth, and stay ahead of industry trends. His insights into analytics and communication empower companies to effectively connect with customers and harness data to fuel their success in an ever-evolving digital world.