Big data analytics enables organizations to gain insight into their business operations and find new ways to increase revenue. However, the data sources used to provide these insights are often located in different places and accessing them can be difficult. Data warehousing and data virtualization are two technologies often used for storing and accessing decentralized data sources.
The main benefit of data virtualization is speed-to-market. Building a big data solution using virtualization is a much faster process than building a data warehouse. Reasons being that you don’t need to design and build the ETL to copy the data. Copying the data means more software licenses, more hardware costs, more data governance costs, more data discrepancies, and more ETL flows. Therefore, data virtualization can also save you a lot of money.
Varada is a new big data virtualization solution that enables enterprises to leverage both speed and flexibility in consuming big data.
A Quick Overview of Varada
Varada is a big data virtualization start-up focused on query acceleration and was founded by Roman Vainbrand, David Krakov, and Tal Ben Moshe, veterans of the Dell EMC XtremIO core team.
Varada’s mission is to leverage virtualization in big data as a way to query data from different sources by using a single endpoint. Varada’s platform aims to deliver full control over query performance, flexibility, and costs by accelerating queries directly on top of the customer’s cloud data lake, within the organizations’ Virtual Private Cloud (VPC).
In September 2020, the company announced a $12 million Series A round of funding. The round was led by MizMaa Ventures, and existing investors F2 Venture Capital, StageOne Ventures, and Lightspeed, who contributed a $7.5 million seed round in early 2019.
The Problem Varada Solves
Organizations are experiencing exponential growth in the amount of data generated and its complexity. However, most of the data’s business value is unused due to the heavy investment in data preparation, data infrastructure, and streamlining data access.
Also, the architecture of most current data infrastructures is based on the traditional approach of data warehouses. In order to meet business requirements of performance, data platform teams are challenged with modeling the data so that it is highly optimized for specific queries. While data is modeled and aggregated, it loses from its granular form which makes agility near impossible. In the cloud era, business needs change extremely fast, leaving data warehouses without the ability to support fast time-to-insights and the required flexibility.
Data Lake Storage is Challenging
With the adoption of cloud computing, data lakes are becoming the leading solution for enterprise data storage and analytics projects. Data lakes are highly scalable storage systems that keep structured and unstructured data in their original form. A data lake does not require users to know the analyses they want to perform; instead, it assumes that breakdown will happen later, on-demand.
However, using data lakes to build data products is challenging, requiring a constant trade-off between cost-effectiveness and time to market. Additionally, querying data from disparate data sources puts a heavy burden on IT operations teams. They need to constantly configure, model, and transfer data. While typical data virtualization can solve these operations problems, performance and cost limitations restrict existing data virtualization tools, as they are often based on compute-heavy brute force for processing and accelerating queries. This is where Varada comes into the picture. It strives to solve both performance limitation issues and unnecessary data preparations to ensure time-to-insights is as fast as possible.
How Varada Revolutionized Data Virtualization
The heart of Varada’s data virtualization platform is inline indexing technology. This technology uses machine learning to understand when users run specific queries and how queries behave and consume resources. Varada uses this data to identify which queries to accelerate and which indexes to maintain. Query prioritization enables users to define and analyze datasets of interests within a data lake using any ANSI SQL, including popular business intelligence tools without worrying about modeling or pre-processing.
Varada runs in the customers Amazon Web Services (AWS) VPC and embeds an open-source Presto SQL query engine to easily connect to any data source. Presto provides an ANSI SQL client and a native method for loading data from multiple data sources. In addition to SQL query distribution, Presto has a thriving community that continues to improve the product.
Varada Solutions
Varada’s dynamic and adaptive big data indexing solution enables users to balance the performance and cost of data applications, BI dashboards, and text analytics solutions.
Data Applications
The extensive processing and complexity of big data analytics applications create a significant challenge in fully monetizing data assets. Varada’s data virtualization technology acts as an intelligent acceleration layer on the data lake. The virtualization layer runs in the customer’s cloud environment and remains a single source of truth. While doing so, Varda makes data accessible to operations teams without them having to transfer, model, or manually optimize data. Varada also enables any SQL query to meet various concurrency and performance requirements while predicting and controlling the costs.
BI & Analytics
Business users often need to view enriched multi-dimensional data across long periods of time. As a result, analytics teams are struggling to keep BI systems and dashboards interactive. Importing limited datasets into internal BI tools is not enough, it can create problems with data freshness. Additionally, limited datasets can restrict concurrency consistency, data volume, and the ability to scale. Varada solves this by integrating with any SQL BI tool to enable queries to run on the entire data lake at a predictable cost, without sacrificing interactivity.
Text Analytics
Text analytics is a vast domain, ranging from log analysis to sentiment analysis. Text analytics enables organizations to access and analyze text-based content such as chats, blogs, logs, metrics, and APM.
Varada supports text analytics directly on the data lake, without the need to move data or build a separate and optimized stack. Using integrated Apache Lucene indexing accelerates text analytics queries. Accelerated analytics enables data professionals to leverage text filters without SQL optimizations or SQL performance tuning. Data teams can also easily integrate text search into BI systems and dashboards.
Takeaway
Some may compare Varada to Snowflake; however, the data virtualization start-up takes a different approach. Varada does not require users to move their data into a third-party vendor t. Customers get to keep their data ownership, data is not copied, and it is not moved, not to mention the advantages of big data indexing which makes queries run much faster at a fraction of the cost
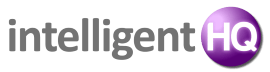
Founder Dinis Guarda
IntelligentHQ Your New Business Network.
IntelligentHQ is a Business network and an expert source for finance, capital markets and intelligence for thousands of global business professionals, startups, and companies.
We exist at the point of intersection between technology, social media, finance and innovation.
IntelligentHQ leverages innovation and scale of social digital technology, analytics, news, and distribution to create an unparalleled, full digital medium and social business networks spectrum.
IntelligentHQ is working hard, to become a trusted, and indispensable source of business news and analytics, within financial services and its associated supply chains and ecosystems